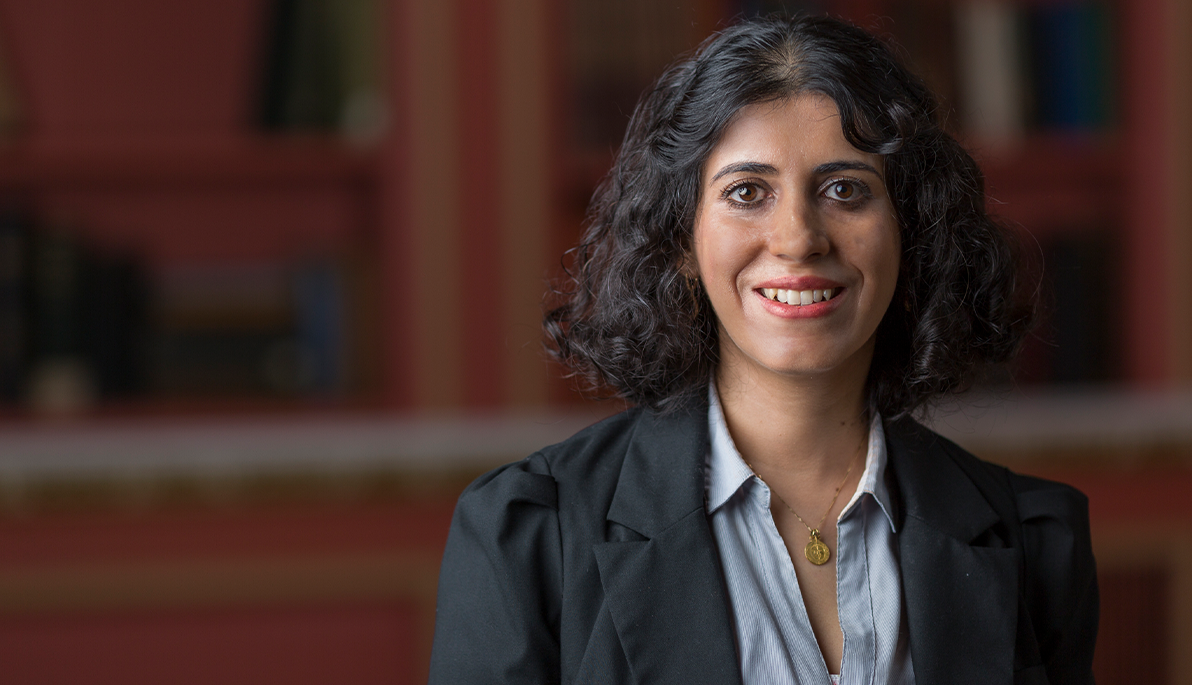
“Engineering” Solutions for Healthier Brains
October 24, 2022
Pictured: Assistant Professor Maryam Ravan, Ph.D.
Could artificial intelligence help solve the mental health crisis? What if an algorithm allowed neurologists to know the area affected by a brain seizure?
These are just two of the questions that Maryam Ravan, Ph.D., assistant professor of electrical and computer engineering, aims to address in new research studies published this fall. The studies, which have been featured in journals and conferences of the Institute of Electrical and Electronics Engineers (IEEE), propose cutting-edge algorithms and techniques that could provide noninvasive solutions for clinicians to more effectively treat neurological and psychiatric conditions.
Normal brain activity, including mood and thinking processes, relies on a balance of fast and slow brain waves. Imbalanced brain wave activity is associated with neurological issues, such as epilepsy and psychiatric conditions, like bipolar disorder and major depressive disorder.
One technique that clinicians use to detect brain wave imbalances is electroencephalography (EEG). This diagnostic test uses sensors to measure the electrical activity on a patient’s scalp, which is representative of the brain wave activity taking place underneath. Brain wave data collected through an EEG holds valuable information regarding an individual’s neurological and mental health.
Ravan has partnered with physicians from prestigious institutions, including Stanford University and McMaster University (Canada), to propose new, non-invasive diagnostic techniques—founded on algorithms and engineering principles—that could improve how physicians use EEG brain wave data to treat the brain.
Locating the Source of a Seizure
Approximately three million adults and 470,000 children in the United States have epilepsy, a neurological condition that causes seizures. During a seizure, the brain’s normal electrical pattern is disrupted, and these sudden bursts of electrical energy can affect consciousness, movements, and sensations.
However, precisely identifying the region of the brain where a seizure originated (brain source) is challenging. Existing software models that process EEG brain wave data use a mathematical technique called exact low-resolution brain electromagnetic tomography (eLORETA). While these models can provide a brain source estimate, they have a high margin of error and do not account for many variables, including individual head shape, which impacts the distribution of electrodes, or the brain’s “electricity producers.” While magnetic resonance imaging (MRI), which does account for head shape, can be used to identify the brain source, these systems are costly, and not every patient can undergo an MRI scan.
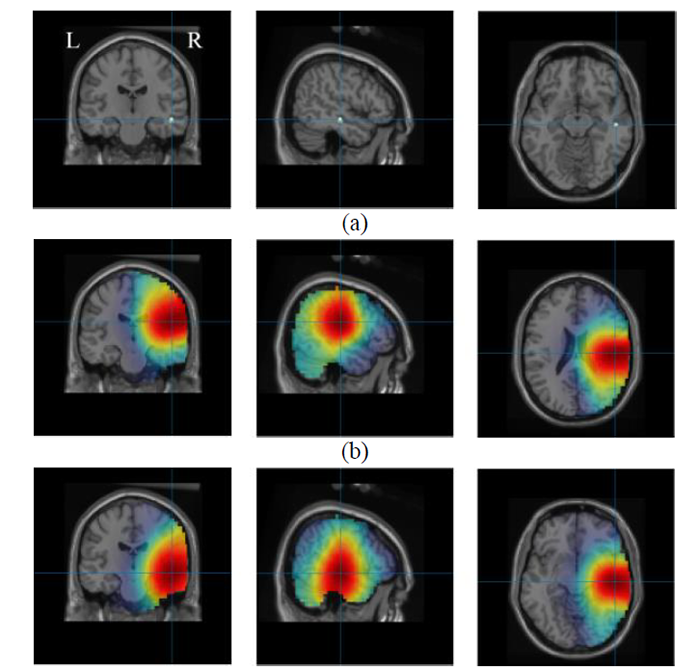
ReLORETA (row c) predicted the true brain source (shown in row a) with more precise estimates than eLORETA (row b).
In a study published September 23 in IEEE Transactions on Biomedical Engineering, Ravan and her fellow co-authors, including Stanford University physicians Robert Fisher, M.D., Ph.D., Babak Razavi, M.D., Ph.D., as well as Amin Noroozi a Ph.D. candidate from Staffordshire University (United Kingdom), propose a more precise and robust brain mapping technique to pinpoint the brain source.
With Ravan’s expertise, the team developed a new algorithm called ReLORETA, which accounts for multiple variables known to impact brain source estimates, including head geometry and misaligned brain electrodes, among other factors. The researchers then applied both ReLORETA and eLORETA to two sets of data: simulated EEG data and actual EEG data collected from real patients. In all instances, ReLORETA provided more robust, accurate results. For example, in the brain’s left hippocampus, which plays a major role in learning and memory, LORETA predicted the brain source with a margin of error of 35.09 millimeters, but ReLORETA’s margin of error was only 5.65 millimeters, providing a much more precise estimate.
“In addition to helping neurologists improve the diagnosis and treatment of epilepsy, ReLORETA may provide a valuable resource for clinicians treating mental health conditions like depression and schizophrenia, which are also often diagnosed using EEG,” says Ravan.
Using AI to Improve Mental Health Diagnoses and Treatments
In collaboration with McMaster University psychiatrist Gary M. Hasey, M.D., Ravan has co-authored two studies that leverage machine learning, a form of artificial intelligence (AI), to improve the treatment of mental health conditions.
In one study, which was presented at this year’s 44th Annual International Conference of the IEEE Engineering in Medicine and Biology Society, Ravan helped develop a machine learning algorithm to analyze patients’ brain waves and categorize their patterns as biomarkers for bipolar disorder or major depressive disorder.
An estimated 21 million adults in the United States had at least one major depressive episode in 2020, and seven million American adults currently live with bipolar disorder. Distinguishing between bipolar disorder and major depressive disorder is a critical challenge, as both diseases have no known biomarkers, and patients can present similar symptoms. Given this, patients are diagnosed solely on their symptoms, personal experiences, and family history.
In addition, treatments for these two conditions can differ greatly and the wrong combination of medications may even increase the risk of suicidal ideation. However, if certain brain wave patterns or activity can serve as biomarkers to distinguish one condition from the other, then clinicians may be able to provide patients with more accurate diagnoses and more effective treatments.
While AI is the broad science of replicating human abilities in computers, machine learning is a specific branch of AI that trains computers to recognize patterns within data sets and predict outcomes or assign them to a category. The technique analyzes unspecified data and categorizes it based on repeated patterns and characteristics. The researchers pose that, given its abilities, machine learning could be useful in analyzing a patient’s EEG results and determining whether a patient is experiencing bipolar disorder or major depressive disorder.
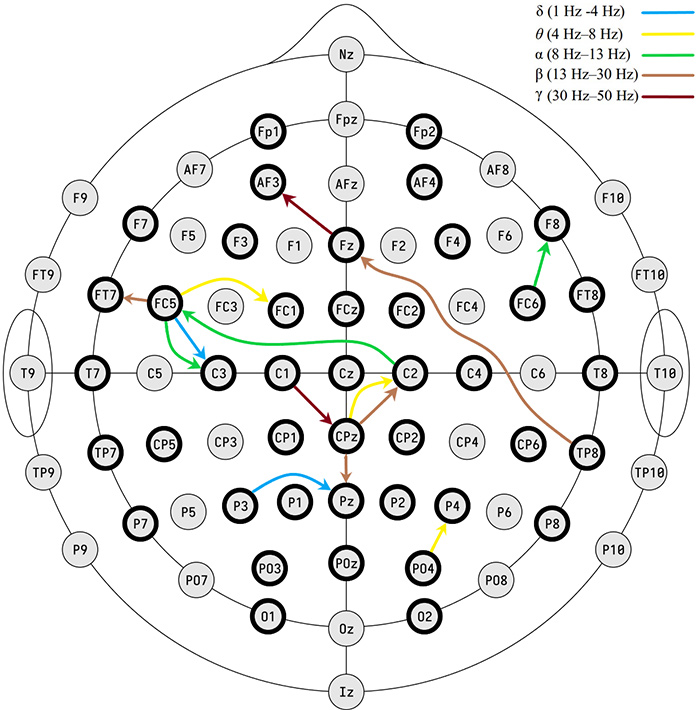
Selected brain wave connectivity features from the study using machine learning to distinguish between bipolar disorder and major depressive disorder. The electrodes highlighted with a bold black circle were used for recording the patients’ EEG signals. δ (1 Hz -3 Hz), θ (4 Hz–7 Hz), α (8 Hz–12 Hz), β (13 Hz–30 Hz), and γ (31 Hz–50 Hz) denote different frequency bands.
To test their theory, Ravan’s new machine learning algorithm was applied to EEG brain wave data collected from 142 patients previously diagnosed with bipolar disorder or major depressive disorder (evenly split). The system then identified data patterns and categorized them as either being associated with bipolar disorder or major depressive disorder. In the end, the technique accurately predicted patients’ real-life diagnoses with more than 83 percent accuracy.
“The accuracy of our algorithm, which was derived from a large sample of patients, successfully distinguished bipolar disorder from major depressive disorder more accurately than the existing methods available to clinicians,” says Ravan. “This suggests that our method may hold significant promise as a clinical tool.”
In addition to Ravan and Hasey, the study’s co-authors include undergraduate students from the College of Engineering and Computing Sciences: Mary Margarette Sanchez, Lee Borden, and Nafiah Alam, as well as Amin Noroozi, a Ph.D. candidate from Staffordshire University (United Kingdom).
Ravan lent her expertise to a second mental health study, which was published on September 12 in IEEE Transactions on Biomedical Engineering. This paper explores how brain wave data could be used to predict how patients with major depressive disorder will respond to the antidepressant sertraline (sold under the brand name Zoloft) vs. a placebo treatment.
While antidepressants are often the first line of treatment for major depressive disorder, it can take months of trial and error and multiple medications before reaching an effective treatment. If predictive biomarkers can inform how a patient will likely respond to a certain treatment, clinicians may be able to eliminate this frustrating process and fast-track a patient’s recovery time.
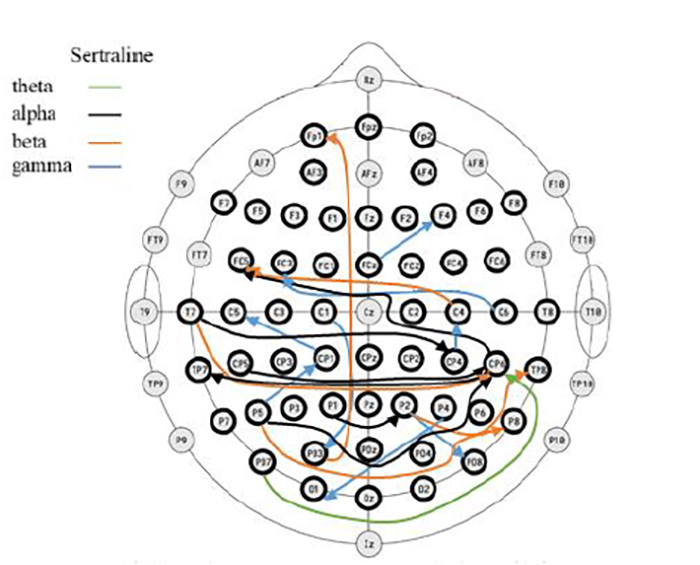
A schematic drawing shows the selected brain wave patterns for predicting response to sertraline at different brain wave frequency bands. Each arrow shows the direction of signals between two EEG electrodes.
Sertraline belongs to a class of antidepressants that increase the levels of serotonin, a chemical messenger in the brain that helps to regulate mood. Serotonin levels also sustain the ratio between slow brain waves, like delta and theta, and faster alpha brain waves. The researchers developed a machine learning algorithm to recognize brain wave patterns and predict whether patients would fare better with sertraline versus a placebo pill. The algorithm was applied to existing NIH EEG brain wave data from 224 patients with major depressive disorder (105 who had received sertraline and 119 who had received the placebo). For each group, the algorithm identified brain wave patterns to discriminate between responders (improved depression symptoms) and non-responders. When compared against the actual patient response data, the algorithm predicted treatment responses with approximately 83 percent accuracy, as also reported by IEEE's news blog IEEE Spectrum.
“By predicting how a patient will respond to various treatments, this technique could provide an inexpensive, non-invasive, and readily available tool for clinicians to more effectively treat their patients, accelerate time to recovery, reduce personal suffering, and decrease treatment costs,” says Ravan.
In addition to Ravan and Hasey, the study’s other co-authors include College of Engineering and Computing Sciences alumni Thomas Oakley (M.S. ’22), Jonathan Coskuner (M.S. ’21), and Andrew Cadwallader (M.S. ’22).
More Features

An Alumnus’ Commitment to the Environment
As an energy management graduate from New York Tech’s Vancouver campus, Jasdeep Gulati (M.S. ’22) is highly invested in educating people about environmental and climate sustainability.

Vancouver Faculty Win University-Sponsored Research Awards in New Program
The new Global Impact Research Grant (GIRG) program has been developed to keep Vancouver-based faculty connected to faculty and research projects being conducted on the university’s New York campuses.

Studying Climate Change One Degree at a Time
Junhua Qu (M.S. ’24) began her collegiate journey in Beijing. But, her interest in climate change took her to New York Tech’s Vancouver campus to study energy management.