Applied Signal and Image Processing Laboratory
The ASIP Lab is focused on research and development in various branches of signal and image processing and analysis, with applications in electrical and biomedical engineering.
LAB DIRECTOR
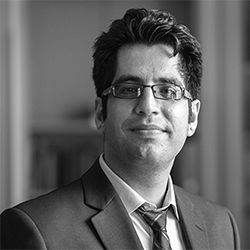
Dr. Ahmadreza Baghaie
- Profile
- Website
- abaghaie@nyit.edu
- Long Island Campus: Harry Schure Hall, Room 222
ACTIVE PROJECTS
Error Quantification in Particle Image Velocimetry
Particle Image Velocimetry as a standard optical imaging technique for flow velocity measurements has gained extensive interest in biomedical applications. In this technique, patient-specific models are 3-D printed using transparent silicon materials for building flow loops, and then realistic flow boundary conditions are administered for the inlets/ outlets of the geometry. The working fluid, which contains micro particles, is imaged using a high-speed multiview camera setup, followed by particle-matching to determine the flow patterns throughout the acquisition.
For an accurate replication of the flow patterns, mechanical properties of the fluid need to match that of the blood. Moreover, optical properties of the silicone model and the fluid also play a significant role in the acquisition and processing of the data. In this project the aim is to study various sources of artifacts in PIV data acquisition and analysis and provide computational tools for modeling and correction of such artifacts.
Deep Learning for Scanning Electron Microscope Data Processing
Scanning Electron Microscopy (SEM) imaging is a crucial technique in various fields of biomedical, mechanical and material sciences for observing surface structure of microscopic samples on a variety of micro-/ nano-meter scales. In SEM a focused beam of electrons scans the surface of the sample while secondary/back-scattered electrons as a result of the interaction of the beam with the surface are captured, signifying the topo- graphical and compositional properties of the surface at any point. SEM data preprocessing involves noise as well as artifact reduction as a means to improve the quality of the captured images. Among the various sources of artifacts, defocus artifacts, which are caused by the high magnification factors and very narrow field-of-view, need to be reduced. On the other hand, SEM micrographs remain two-dimensional, lacking the 3-D surface information. The advent of deep learning and Convolutional Neural Networks (CNN)- based techniques has made significant impact in modern computer vision in recent years.
The project aims to introduce novel deep learning and CNN-based methods to reduce the adverse effects of artifacts and improve the interpretability of the captured 2-D micrographs by 3-D surface reconstruction of the microscopic samples.