Radar and Communications Laboratory
The Radar and Communications (RadCom) lab focuses on developing signal processing, optimization, and machine learning techniques for radar and communications. The lab also focuses on developing physical layer security and authentication methods for wireless networks.
LAB DIRECTOR
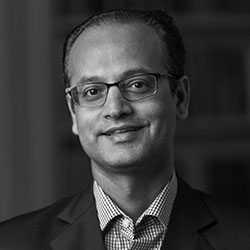
Dr. Batu Chalise
- Profile
- Website
- bchalise@nyit.edu
- Long Island Campus: Harry Schure Hall, Room 212
ACTIVE PROJECTS
Joint Radar and Communications Systems (Supported by NYIT-ISRC)
Due to the ever-increasing number of wireless devices and users, there is a need to optimally utilize the available frequency spectrum. The joint radar communication develops the same platform of transmitters/receivers and functions both as radar and communications. The first objective of this project is to develop optimal resource (e.g., bandwidth, power, antennas, time slots) allocation schemes so that the diverse requirements of both radar and communications receivers are simultaneously satisfied. The second objective is to develop signal processing techniques for embedding information into radar waveforms with and without re-modulation approach.
Passive Radar Systems (Supported by Air Force Research Laboratory)
Passive radar systems (PRS) utilize the broadcast signals from non-cooperative transmitters that are not an integral part of the radar transmitter-receiver set. These transmitters may include radio, television, and cell phone base stations, which are locally available around the radar receiver. As such, PRS do not need dedicated transmitting components, hence lowering both the cost and size of the radar systems. However, in contrast to active radar systems, which have control over transmitters' parameters, such as bandwidth, power, and transmit waveforms, PRS may need to rely on narrowband and low-powered broadcast signals. Moreover, the transmitted signal is not known a-priori at the radar receiver. Due to these reasons, conventional techniques are not directly applicable for PRS. The objective of this research is to develop signal processing, optimization, and machine learning-based techniques for detection and tracking of targets in PRS.
Signal Processing and Security for 5G Systems
5G systems need to support high data rate transmissions under stringent requirements for reliability, coverage, energy consumption, and low latency. The 5G and beyond networks are also expected to support heterogeneous traffic that includes machine-type communications, Internet of Things (IoT), and vehicle to everything. The objective of this research is to develop coding/ decoding, channel estimation, beamforming/precoding, scheduling, interference management, and resource allocation methods leveraging concepts on massive multiple-input multiple-output, mmWave, full-duplex, non-orthogonal multiple access (NoMA), and edge computing. The development of deep learning techniques for solving N-P hard resource allocation problems and ensuring secure authentication are also the key objectives of this research.
EQUIPMENT
- Aronia Spectrum analyzer (up to 20 GHz)
- Rigol Signal generator (up to 6 GHz),
- Two Tektronix Mixed Domain Digital Oscilloscopes
- Three USRP-2901 kits from National Instruments
- A 3x3 MIMO CSI collection setup with Intel NIC 5300 and a router with 3 antennas
SAMPLE PUBLICATIONS
- S. Guruacharya, B. K. Chalise, and B. Himed, "MAP ratio test detector for radar system," IEEE Transactions on Signal Processing, in press, Dec. 2020.
- S. Guruacharya, B. K. Chalise, and B. Himed, "Off-Grid compressed sensing for radar with unknown transmit waveform," IEEE International Radar Conference, Washington D.C., Apr.–May 2020.
- S. Guruacharya, B. K. Chalise, and B. Himed, "Energy distribution of multiple target signal with application to target counting," IEEE Signal Processing Letters, vol. 27, pp. 431–435, Feb. 2020.
- S. Guruacharya, B. K. Chalise, and B. Himed, "On the product of complex Gaussians with application to radar," IEEE Signal Processing Letters, vol. 26, no. 10, Oct. 2019.
- S. Pak, B. K. Chalise, and B. Himed, "Target localization in multi-static passive radar systems with artificial neural networks," in International Radar Conference, Toulon, France, Sept. 2019.